My opinions for the exs:
I’ m not so sure about ex.7and ex.9 can anybody help me?
ex.1
1.Initialize the w to zeros.
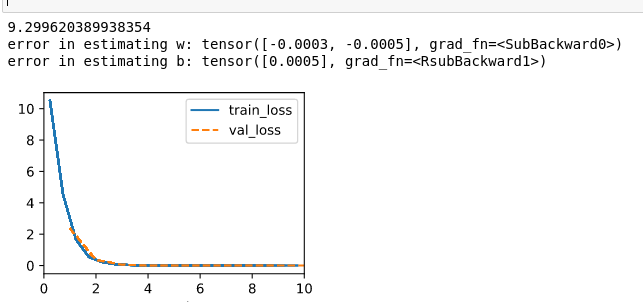
2. Initialize w by norm distribution.
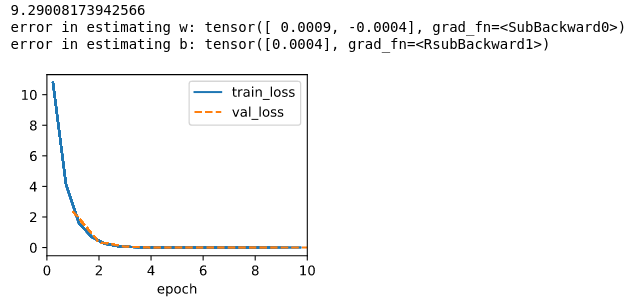
3. Initialize the w by norm distribution with sigma set to 1000
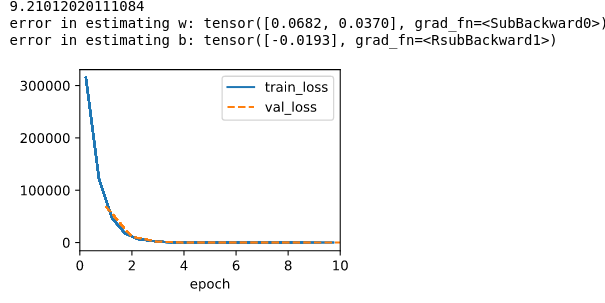
Note that I set max epoch to 10, seems like if I start w with all zero, it doesn’t make any difference, but if I change the sigma from 0.01 to 1000, the training speed doesn’t change, but the training error start with a huge quantity, and ends with a number increase by two orders of magnitude for both w and b.
ex.2
Step1 I produce a class to generate the examples composed by self.V = voltage, self.I = current, self.IR = I*R
%matplotlib inline
import torch
from d2l import torch as d2l
import random
class SyntheticRegressionData_202209031839(d2l.DataModule):
def __init__(self, r_list, noise=0.1, num_train=100, num_val=100, batch_size=32, shuffle=True):
super().__init__()
self.save_hyperparameters()
#for every resistor r, send some voltage and get some current
#assume that the voltage is the range(1,num_train+1,1)
V_train = torch.tensor([])
V_val = torch.tensor([])
I_train = torch.tensor([])
I_val = torch.tensor([])
IR_train = torch.tensor([])# to save the value of I*R
IR_val = torch.tensor([])
for r in r_list:
#V_train_tmp=torch.abs(torch.randn(num_train, 1))
V_train_tmp = torch.tensor(range(1,num_train+1,1)).reshape(-1,1)
V_train = torch.cat((V_train, V_train_tmp), 0)
I_train_tmp = V_train_tmp/r + torch.randn(num_train, 1) * noise
I_train = torch.cat((I_train, I_train_tmp), 0)
IR_train = torch.cat((IR_train, I_train_tmp*r), 0)
for r in r_list:
V_val_tmp = torch.tensor(range(1,num_val+1,1)).reshape(-1,1)
V_val = torch.cat((V_val, V_val_tmp), 0)
I_val_tmp = V_val_tmp/r + torch.randn(num_val, 1) * noise
I_val = torch.cat((I_val, I_val_tmp), 0)
IR_val = torch.cat((IR_val, I_val_tmp*r), 0)
#need to be suffle or the examples will be ordered by the r value
indices_train = list(range(0, self.num_train*len(r_list)))
indices_val = list(range(0, self.num_train*len(r_list)))
if shuffle:
random.shuffle(indices_train)
random.shuffle(indices_val)
#combine examples for training and validating
self.V = torch.cat((V_train[indices_train], V_val[indices_val]), 0)
self.I = torch.cat((I_train[indices_train], I_val[indices_val]), 0)
self.IR = torch.cat((IR_train[indices_train], IR_val[indices_val]), 0)
Step2 As I experimented with 2 different resistors, each one will be put voltage(I) vary from 1 to 100, and I evaluate the current(I), and plot them, judging from the image, the relation between I and V of the same resistor is linear.
import matplotlib.pyplot as plt
data_1= SyntheticRegressionData_202209031839([1])
data_2= SyntheticRegressionData_202209031839([2])
plt.plot(data_1.V, data_1.I, 'o', data_2.V, data_2.I, 'o')
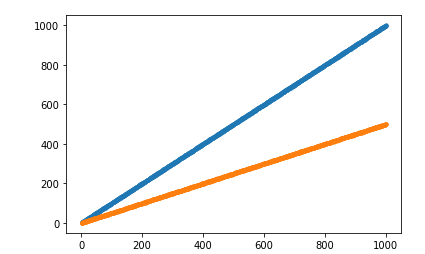
Step3 Define the data loader.
@d2l.add_to_class(SyntheticRegressionData_202209031839)
def get_dataloader(self, train):
#the real num_train is num_train*len(self.r_list)
i = slice(0, self.num_train) if train else slice(self.num_train, None)
return self.get_tensorloader((self.IR, self.V), train, i)
Step4 Train the model, learning rate is suggested to set to a small value, or it will get many NAN at the forward phase, and the conclution is that U = I*R.
data= SyntheticRegressionData_202209031839(r_list = range(1,11,1))
model = LinearRegressionScratch(1, lr=0.0003, sigma = 0.01)
trainer = d2l.Trainer(max_epochs=3)
trainer.fit(model, data)
print(f'error in estimating w: {1. - model.w}')
print(f'error in estimating b: {0. - model.b}')
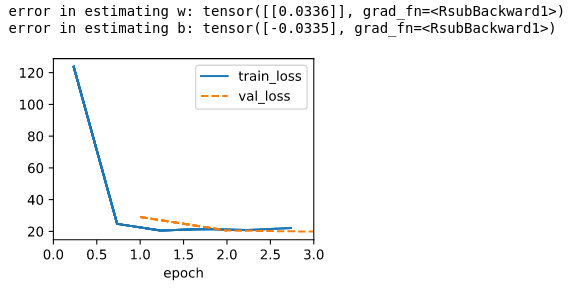
ex.3
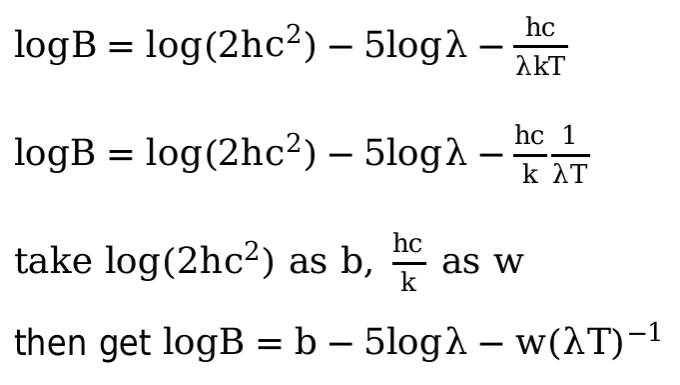
As the procedure shown in the picture, I think during the experiment, the λ, B, T can be preprocessed as logB, -5logλ, (λT)^-1, where logB will be the y, -5logλ and (λT)^-1 will be x1 and x2. Then the problem become a linear regression, after we have train out the w and b, we can use it to compute T with a B and λ.
ex.4
I tested with a simple linear function.
def loss(y_hat, y):
l = (y_hat - y.reshape(y_hat.shape)) ** 2 / 2
return l.mean()
x = torch.tensor([[1.,2.],[3.,4.]])
b = torch.tensor([1.]).requires_grad_(True)
w = torch.tensor([1.,2.]).reshape(-1,1).requires_grad_(True)
y_hat = (torch.matmul(x, w) + b)
y = torch.tensor([5.,6.])
loss = loss(y_hat, y)
loss.backward()
print(w.grad, b.grad)
loss.backward()
print(w.grad, b.grad)
The first backward works well , and the grad is tensor([[ 9.5000], [13.0000]]) tensor([3.5000])
But the second backward one cause a error:
RuntimeError: Trying to backward through the graph a second time (or directly access saved tensors after they have already been freed). Saved intermediate values of the graph are freed when you call .backward() or autograd.grad(). Specify retain_graph=True if you need to backward through the graph a second time or if you need to access saved tensors after calling backward.
As the ouput say, I should add “retain_graph=True” at the first backward() or the grad will be drop each automatically. So I change the code at the first backward to
loss.backward(retain_graph=True)
and the output is:
tensor([[ 9.5000], [13.0000]]) tensor([3.5000])
tensor([[19.], [26.]]) tensor([7.])
ex.5
I think because the element-wise subtraction can only be used between two tensors with same shape, unless one of them is a scalar.
ex.6
I use the same synthetic data in this chapter, test lr = [0.003, 0.03, 0.3, 3] with max_epoch = 3, and find that 0.03 is too small that the reducing of error(both train and val) is too slow, 3 is too large that the error keep growing as the epoch proceed, 0.3 is the fast one that reach 0 error before the first epoch.
Then I use max_epoch = 10 to see what’s happen, it works well for the lr=0.003, but still not enough, and makes little difference to lr=0.3 and lr=0.03 cause I think they have already reached a tiny error within 3 epochs, and besides, the error of lr=3 still growing.
May be lr =3 is too large compared to the scale of w and b.
ex.7
I added the code below into the function fit_epoch(), and can see some 8 in the output.
if batch[0].shape[0] != 32:
print(batch[0].shape[0])
So maybe the data_iter just give out all the examples out to train or validate when the number can’t match the batch size.
ex.8
A I tested with those two code snippets below, while the data keeps the same, seems like the training drops sharply at the beginning but then floating near a error that is not that ideal, but the validate eroor is always small than the train error.
@d2l.add_to_class(LinearRegressionScratch) #@save
def loss(self, y_hat, y):
l = (y_hat - d2l.reshape(y, y_hat.shape)).abs().sum()
return l.mean()
model = LinearRegressionScratch(2, lr=0.03, sigma = 0.01)
data = d2l.SyntheticRegressionData(w=torch.tensor([2, -3.4]), b=4.2)
trainer = d2l.Trainer(max_epochs=10)
trainer.fit(model, data)
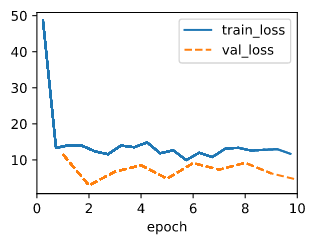
B I use this code to perturb,
data.y[4] = 10000
The output for squared loss is:
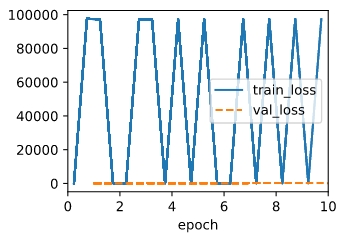
The output for abs loss is:
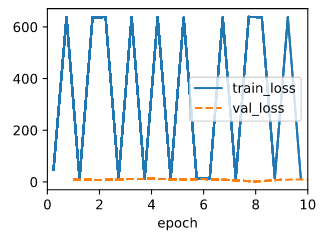
Judging from the result, seems like the squared loss is more vulnerable to large value.
C The abs loss is fast and robust to large value, the squared loss is more accurate.
Maybe I can use the sqrt of the mean squared error.
@d2l.add_to_class(LinearRegressionScratch) #@save
def loss(self, y_hat, y):
l = (y_hat - y.reshape(y_hat.shape)) ** 2
l = l.sum().mean().pow(0.5)
return l
And the test:
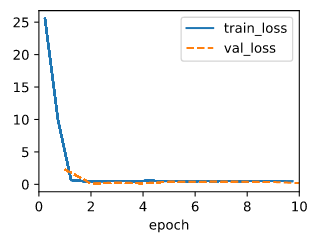
model = LinearRegressionScratch(2, lr=0.03, sigma = 0.01)
trainer = d2l.Trainer(max_epochs=10)
data = d2l.SyntheticRegressionData(w=torch.tensor([2, -3.4]), b=4.2)
trainer.fit(model, data)
ex.9
I think it’s to break the relation between the data because we don’t need that information.
The shuffle fuction in random package is still run under a predictable formula, so the shuffle is also not that reliable(? I’m not so sure about that).
Like the code I used in ex.2 to produce voltage and current, if I don’t use shuffle, the data will be arranged in the order of the resistor, in other words, the voltage and current get from the same resistor is near to each other.